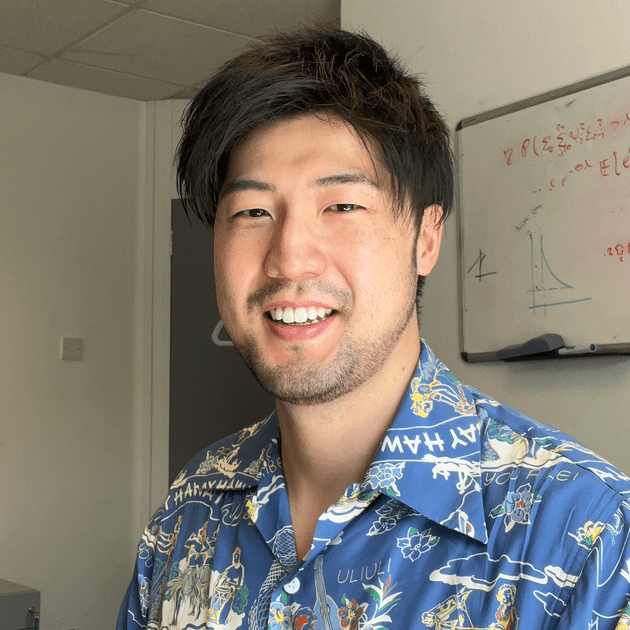
Motonori Oka (岡 元紀)
Motonori Oka (岡 元紀)
Motonori Oka (岡 元紀)
About Me
Hi, I'm Motonori Oka (岡 元紀).
I am pursuing a PhD in Statistics at the Social Statistics Group in the Department of Statistics at the London School of Economics and Political Science (LSE). I am very fortunate to have the great opportunity to do my PhD research under the supervision of Yunxiao Chen and Irini Moustaki in the Psychometrics Lab.
Prior to the LSE, I received an M.A. in Education (2022) from the University of Tokyo and a B.A. in Psychology (2019) from University of Tsukuba, Japan.
My primary research interests lie broadly in statistics and machine/deep learning for the education and social sciences, with specific devotion to methodological advancement in latent variable modeling that contributes to the better understanding of the complex phenomena behind human behaviors. The thrust of my research has been centered on scalable psychometrics, education data science, and statistics education. In particular, I am interested in scalable estimation algorithms and their theory of latent factor models. My goal is to contribute to the development of scalable psychometrics through methodological and applied research on latent variable modeling in large-scale settings, such as online learning platforms, crowdsourcing, and international large-scale assessments in education.
I am also actively engaged in collaborating with educational and psychological researchers to help their decision making in their applied research.
Publications
Referred Articles
- Oka, M., & Okada, K. (2022). Scalable Bayesian approach for the DINA Q-matrix estimation combining stochastic optimization and variational inference. Psychometrika. https://link.springer.com/article/10.1007/s11336-022-09884-4
(Its arXiv version is available at https://arxiv.org/abs/2105.09495) - Oka, M., Saso, S., & Okada, K. (2022). Variational Bayesian inference for a polytomous-attribute saturated diagnostic classification model with parallel computing. Behaviormetrika. https://doi.org/10.1007/s41237-022-00164-0
(Its arXiv version is available at https://arxiv.org/abs/2107.01865)
IN JAPANESE
- Saso, S., Oka, M., & Usami, S. (2024). Examining estimation accuracy of cognitive diagnostic models in classroom contexts: Focusing on the impact of model misspecification and different estimation methods. Proceedings of the Institute of Statistical Mathematics in Japan. (in Japanese)
(原文)佐宗駿・岡元紀・宇佐美慧 (2024). 小サンプルサイズ下での認知診断モデルの推定精度の検討:モデルの誤設定の影響と推定法の違いに着目して. 統計数理. - Saso, S., Oka, M., & Uesaka, Y. (2023). Diagnosing the depth of understanding using cognitive diagnostic models and their application to a regular test: Examining the applicability of cognitive diagnostic models with a Q-matrix specified through qualitative and quantitative procedures. Cognitive Studies: Bulletin of the Japanese Cognitive Science Society. (in Japanese)
(原文)佐宗駿・岡元紀・植阪友理 (2023). 認知診断モデルを活用した理解の深さの診断と定期テストへの応用:定性的・定量的なQ行列の設定とモデルの実践的有用性の検討. 認知科学.
Papers Submitted
- Oka, M., Chen, Y., & Moustaki, I. (2024). Learning high-dimensional latent variable models via doubly stochastic optimisation by unadjusted Langevin. Submitted to Journal of Educational and Behavioral Statistics.
(Its arXiv version is available at https://arxiv.org/abs/2406.09311) - Hijikata, K., Oka, M., Yamaguchi, K., & Okada, K. (2024). variationalDCM: An R package for variational Bayesian inference in diagnostic classification models. Submitted to Journal of Statistical Software.
(Its PsyArXiv version is available at https://psyarxiv.com/f2sqd/)
Preprints
- Oka, M., & Okada, K. (2021). Assessing the performance of diagnostic classification models in small sample contexts with different estimation methods. arXiv. https://arxiv.org/abs/2104.10975
Presentations
Invited Presentations
- Hijikata, K., Oka, M., Yamaguchi, K., & Okada, K. (2023). Variational Bayesian estimation in diagnostic classification models [Oral presentation]. The 6th International Conference on Econometrics and Statistics (EcoSta) 2023 [Session: Latent variable models and applications], Tokyo, Japan.
- Okada, K., Fukushima, K., & Oka, M. (2022). Diagnostic classification modeling for making the most use of item response data [Oral presentation]. Japanese Joint Statistical Meeting 2022 JSS-KSS-CSA [Session: Complex data analysis], Tokyo, Japan.
- Saso, S., Oka, M., Shiba, S., & Uesaka, Y. (2022). The practical application of cognitive diagnostic models to encourage students’ deep understanding and use of effective learning strategies [Oral presentation]. The 11th Conference of the Asian Regional Section of the International Association for Statistical Computing (IASC-ARS) 2022 [Session: Recent developments in cognitive diagnostic models], Kyoto, Japan.
Referred Presentations
- Oka, M., Chen, Y., & Moustaki, I. (2024). Estimating high-dimensional latent variable models via minibatch variance-reduced stochastic optimisation [Oral presentation]. International Meeting of Psychometric Society (IMPS) 2024, Prague, Czech.
- Okada, K., Hijikata, K., & Oka, M. (2024). Bayesian joint modal estimation for sparse item factor analysis [Oral presentation]. International Meeting of Psychometric Society (IMPS) 2024, Prague, Czech.
- Oka, M., Chen, Y., & Moustaki, I. (2023). A doubly stochastic gradient algorithm for high-dimensional latent variable models [Oral presentation]. International Meeting of Psychometric Society (IMPS) 2023, Washington, D.C., USA.
- Hijikata, K., Oka, M., & Okada, K. (2023). Sparse Bayesian joint modal estimation for item factor analysis [Oral presentation]. International Meeting of Psychometric Society (IMPS) 2023, Washington, D.C., USA.
- Saso, S., Oka, M., & Uesaka, Y. (2023). Development of assessment tools for depth of understanding quantitatively with cognitive diagnostic models [Poster presentation]. Future of Information and Communication Conference (FICC) 2023, Online.
- Hijikata, K., Oka, M., Yamaguchi, K., & Okada, K. (2022). The development of "variationalDCM" an R package performing variational Bayesian estimation for DCMs [Oral presentation]. The 15th International Conference of the ERCIM WG on Computational and Methodological Statistics (CMStatistics) 2022, London, United Kingdom.
- Saso, S.†, Oka, M.†, & Uesaka, Y. (2021). How can we statistically gauge students’ deep understanding from high school regular tests? [Poster presentation]. Junior Researchers of European Association for Research on Learning and Instruction (JURE) 2021, Online.
(†equal contribution) - Oka, M., & Okada, K. (2021). New iterative algorithm for the DINA Q-matrix estimation [Oral presentation]. International Meeting of Psychometric Society (IMPS) 2021, Online.
- Oka, M., Saso, S., & Okada, K. (2021). Parallelized variational Bayesian algorithm for the polytomous-attribute saturated diagnostic classification model [Oral presentation]. World Meeting of the International Society for Bayesian Analysis (ISBA) 2021, Online.
IN JAPANESE
- Saso, S., Oka, M., & Uesaka, Y. (2022). Application of cognitive diagnostic models for quantitative assessment of depth of understanding and its proposed process: Q-matrix design utilizing the findings of psychology and mathematical education and Q-matrix estimation [Poster presentation]. The 39th Annual Meeting of Japanese Cognitive Science Society, Tokyo, Japan. (in Japanese)
(原文)佐宗駿・岡元紀・植阪友理 (2022). 理解の深さの定量的評価を目指した認知診断モデルの応用とそのプロセスの提案–心理学・教科教育の知見とQ行列推定を活かしたQ行列作成– [ポスター発表]. 日本認知科学会第39回大会, 東京, 日本.
Non-Referred Presentations
IN JAPANESE
- Hijikata, K., Oka, M., & Okada, K. (2023). An R package "variationalDCM" for variational Bayesian estimation in dignostic classification models [Oral presentation]. The 51th annual meeting of the Behaviormetric Society, Tokyo, Japan. (in Japanese)
- Saso, S., Oka, M., Shiba, S., & Uesaka, Y. (2022). Quantitatively evaluating the degree of student's understanding and instructing learning strategies: Practical applications of cognitive diagnostic models and student's responses [Poster presentation]. The 20th conference of the Japan Association for Research on Testing, Online. (in Japanese)
- Oka, M., & Okada, K. (2021). Learning latent structures in Q-restricted latent class models with a non-compensatory assumption [Oral presentation]. Japanese Joint Statistical Meeting 2021 Competition Session, Online. (in Japanese)
- Saso, S., Oka, M., & Uesaka, Y. (2021). An empirical analysis of deep understanding through cognitive diagnostic models: Analysis using a large-scale standardized achievement test and its comparison with traditional perspectives [Oral presentation]. The 19th conference of the Japan Association for Research on Testing, Online. (in Japanese)
- Saso, S., Oka, M., & Uesaka, Y. (2021). Measuring deep understanding and its application to regular examinations: Applying cognitive diagnostic models to teaching practice and the responses from classroom teachers [Oral presentation]. Spring conference 2021 of the Japan Society of Educational Technology, Online. (in Japanese)
- Oka, M., & Okada, K. (2020). Assessing the performance of Q-matrix estimation in a large-scale assessment [Oral presentation]. Young Researchers’ Seminar 2020 of the Japanese Society of Computational Statistics, Online. (in Japanese)
- Oka, M., & Okada, K. (2020). Q matrix estimation algorithm using variational inference and stochastic optimization [Oral presentation]. The 48th annual meeting of the Behaviormetric Society, Tokyo, Japan. (Conference canceled)
- Oka, M., & Okada, K. (2020). Q matrix estimation algorithm combined with variational inference and sampling technique [Oral presentation]. The 34th conference of the Japanese Society of Computational Statistics, Online. (in Japanese)
Review
- Psychometrika (2024~)
- British Journal of Mathematical and Statistical Psychology (2024~)
- Oka, M., & Okada, K. (2022). Scalable Bayesian approach for the DINA Q-matrix estimation combining stochastic optimization and variational inference. Psychometrika. https://link.springer.com/article/10.1007/s11336-022-09884-4
Awards and Honors
2024
Scholarship for Postgraduate Study
Japan Student Services Organization
2023
Duolingo Travel Award
Duolingo & Psychometric Society
2022
Scholarship for Postgraduate Study in UK
Keidanren Ishizaka Memorial Foundation & The Tokyo Club
2021 (offered/declined)
Research Fellowship for Young Scientists (DC1)
The Japan Society for the Promotion of Science (JSPS)
2020
Student Research Presentation Award
The 34th conference of the Japanese Society of Computational Statistics
Contact
EMAIL: m.oka1[at]lse.ac.uk
Please replace [at] with @ when you send an email to me.